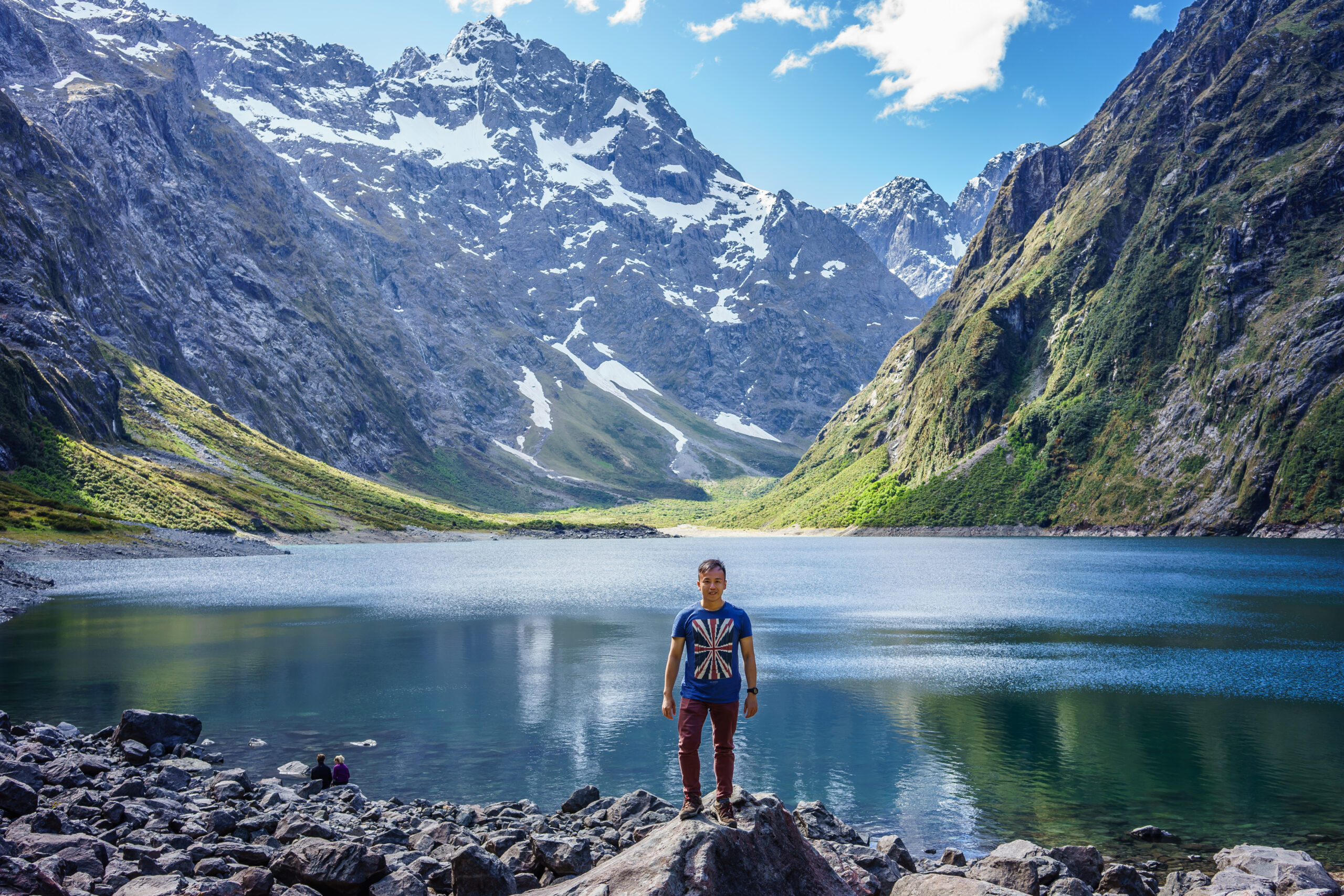
Scholar-Elect Ping Lin Yeap talks about how his PhD will investigate the role of machine and deep learning in reducing errors in cancer scans.
This research has the potential to revolutionise adaptive radiotherapy workflows in clinical settings and contribute to the advancement of personalised and precision medicine
Ping Lin Yeap
The aim of Ping Lin Yeap’s research is to “create a Netflix for cancer treatment”, he says. By that he means that he wants to personalise cancer treatment, ensuring that it is able to adapt a patient’s radiotherapy plan based on how their anatomy changes on any given day.
Cancer can lead to weight loss and other anatomical changes, which can affect tumour dose and cause side effects in healthy tissues. Ping Lin’s PhD in Oncology, which he begins in the autumn, hopes to draw on data from Cambridge and his home country of Singapore to make it possible to apply computational methods to enhance CT scans taken during cancer treatment. This enables a more accurate determination of the delivered dose to individual patients and allows for side effects to be minimised.
Growing up in Singapore
Ping Lin [2023] was born in Singapore. Influenced by his parents who both studied engineering, he was naturally inclined towards STEM subjects at school and represented his school in international mathematics competitions. As he approached the end of his schooling, he remained uncertain about his future path, except for two desires: to pursue physics and to study abroad in order to gain a broader worldview. Having done A Levels and having been inspired by Stephen Hawking’s books, it seemed logical to apply to physics courses in the UK. Ultimately, he chose Imperial College, envisioning London as an ideal launchpad for exploring Europe.
Undergraduate studies at Imperial College
While studying at Imperial, Ping Lin met his partner. Her parents had passed away from cancer at a relatively young age, and that sparked a desire to pursue medical physics. In his third year, he participated in a research project that explored the application of protons in radiotherapy for cancer treatment. Traditionally, radiotherapy relied on high-energy photons and electrons, but advancements in technology have introduced the use of protons and ions in the field. Ping Lin’s primary objective was to improve an analytical model that could predict the energy deposition of proton beams in water, enabling an investigation into how the thermal energy generated could induce double strand breaks in DNA. “Ultimately, I aimed to develop a deeper understanding of proton beam therapy from a physics perspective,” he explains.
Master’s studies at Cambridge
Ping Lin subsequently moved to Cambridge where he pursued his Master of Philosophy (MPhil) in Physics at the Cavendish Laboratory. As part of the VoxTox computational radiotherapy group, his research focused on adaptive radiotherapy for head-and-neck cancer patients. For his MPhil project, he developed an automatic contouring script using deformable image registration to compare the discrepancy between planned and actual delivered dose in a cohort of patients treated at Addenbrooke’s Hospital, and correlated the delivered dose to the spinal cord with Lhermitte’s Sign toxicity.
His work demonstrated the benefits of adapting radiotherapy treatment plans to accommodate changes in a patient’s anatomy and weight. “It’s a more precise and personalised approach,” he says. “The gold standard would be to personalise a patient’s radiotherapy plan to account for both spatial and temporal variations, so there are minimal side effects while maximising damage to the tumour.”
Public service in Singapore
As the Singapore government covered the expenses for his four-year study abroad, Ping Lin was obliged to return to Singapore upon completing his master’s degree. He began his career by teaching Physics at a public school for three years. He then transitioned to the Ministry of Education headquarters, where he worked on the development of continuing education policies. During his tenure there, Ping Lin undertook a range of assignments, including legislation amendment and the implementation and analysis of a nationwide survey. He is passionate about promoting equitable access to STEM education and, in his free time, he volunteered at a community programme that offered free coding lessons to underprivileged young people, who would otherwise not have been exposed to computational thinking skills. This combination of social leadership and academic excellence played a pivotal role in securing him a Gates Cambridge scholarship.
After six years, Ping Lin decided to return to the field of radiation oncology. He joined the National Cancer Centre Singapore, working with a team of oncologists, physicists and therapists to deliver optimal treatment to patients. As a medical physicist, he planned radiotherapy treatment plans and commissioned new medical technologies, such as linear accelerators and proton beam gantries. Beyond his clinical duties, Ping Lin mentors undergraduate interns from local universities while also actively engaging in research on AI-guided adaptive radiotherapy. His latest work on a deep learning-based approach to predicting the accuracy of deformably registered contours was published in the prestigious Physics in Medicine and Biology journal.
Advancing adaptive radiotherapy through a PhD
Last year, Ping Lin decided that he wanted to continue his studies and applied to do a PhD at Cambridge. Under the guidance of Dr Raj Jena, his PhD research will evaluate machine learning and deep learning approaches to predict and minimise errors between registered CT images by harnessing the power of artificial intelligence to acquire real-time, comprehensive information about patients’ anatomical changes. Currently, high-quality CT scans are taken weeks prior to treatment for planning purposes, while lower-quality cone-beam CT scans are taken at each treatment session for patient positioning.
However, it can be difficult to discern anatomical details from cone-beam CT scans. To address this, Ping Lin plans to employ deformable image registration techniques, deforming the planning CT scan to align with the fractional cone-beam CT scans. The aim is to facilitate the detection of anatomical and hence dose changes.
In addition to evaluating machine learning and deep learning approaches to reduce errors, Ping Lin, who will return to the team he worked with during his MPhil, is also keen to use generative AI to enhance the quality of cone-beam CT scans, enabling their direct usage for plan adaptation. “This research has the potential to revolutionise adaptive radiotherapy workflows in clinical settings and contribute to the advancement of personalised and precision medicine,” he says.