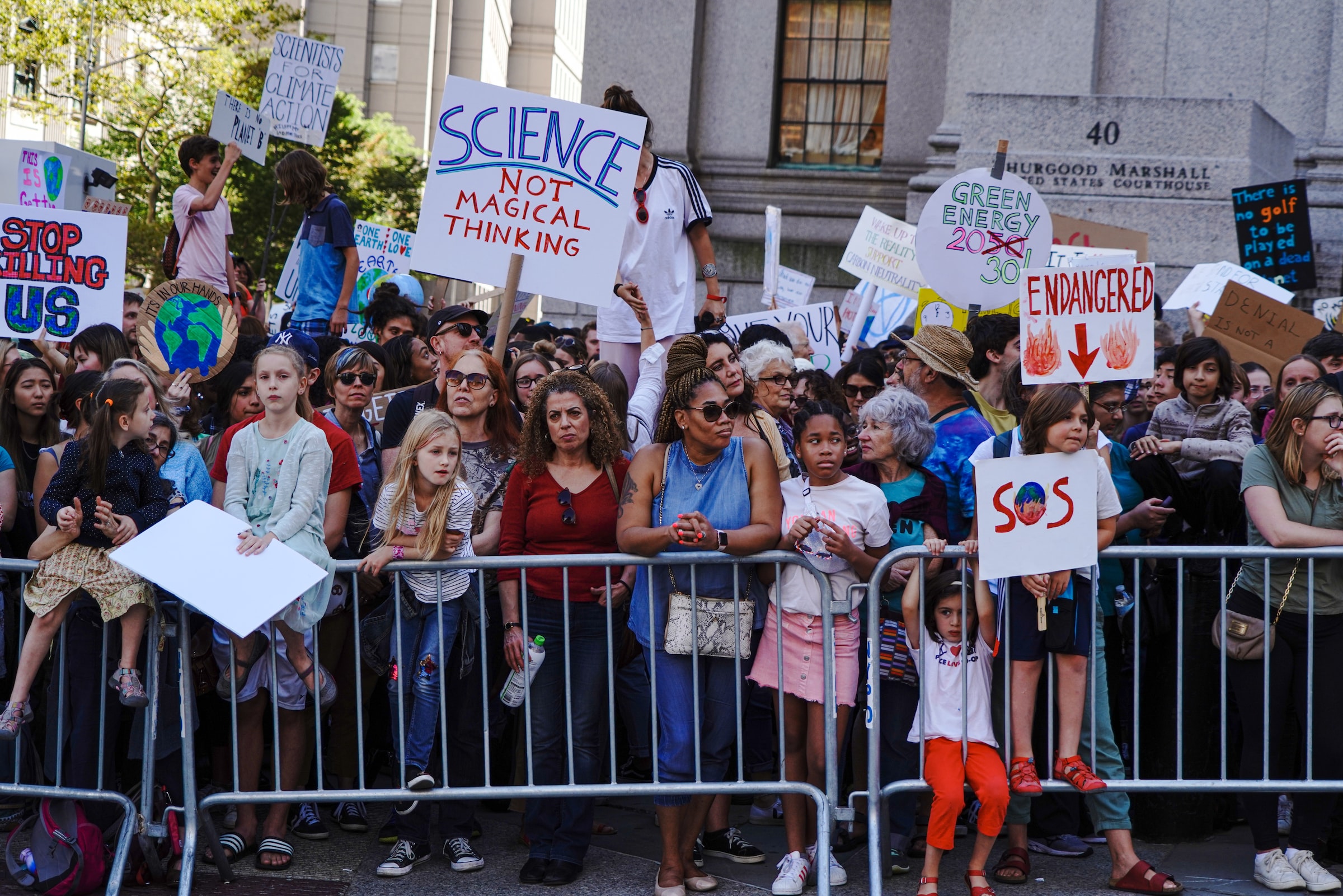
Ramit Debnath is a co-signatory to a letter in Nature Human Behaviour on how to tackle climate change misinformation
Restoring public trust in climate research using behavioural science can be facilitated using large population-scale modelling that produces insights that can drive individual-level attitudinal and behavioural change
Ramit Debnath et al
Future leaders and researchers need to be urgently trained to tackle climate change misinformation through an interdisciplinary approach that foregrounds computational social science and extends beyond laboratories and university campuses to shape the science-policy interface and rebuild public trust in climate research, according to leading academics.
Writing in Nature Human Behaviour, the academics, including Dr Ramit Debnath [2018], a Gates Cambridge Scholar and the first Cambridge Zero Fellow at the University of Cambridge, argue that harnessing the power of computational social science – and behavioural science – in combination with an adequately funded, multidisciplinary and concerted research programme will make it possible to “leverage the promise of big data and behavioural science to encourage appropriate climate action through consensus generation by reducing misinformation, removing scepticism and restoring trust”.
The article is a response to a comment piece that recommended the collection of large-scale behavioural datasets through public data observatories to enable system-level climate action. The authors say that the collection of datasets alone is not sufficient to address the urgency of the climate change issue and that data scientists and social scientists need to collaborate in order to move from data to action.
In addition, they state that such an approach raises “considerable challenges” with regard to the political neutrality of the data, protecting privacy in open datasets, adhering to internationally agreed-upon scientific practices, making the data relevant to policy and sustaining the funding required to collect international and representative cross-sectional and longitudinal surveys.
They argue that computational social science can act as “a critical interdisciplinary bridge” between data science and human behavioural theories, generating key insights using observational, experimental and machine-learning methods, citing, for example, a recent study which identified growing polarisation in public opinions regarding climate change. They say it can extract behavioural insights at scale from the data observatories, enabling systems design that counters misinformation and polarisation and supports consensus building for climate mitigation and adaptation policies.
Despite challenges, which they identify, they say an operationalising framework for aiding system designs could reduce climate misinformation, remove scepticism and recover trust in science through data-driven processes, including clustering, categorisation and forecasting.
They state: “Restoring public trust in climate research using behavioural science can be facilitated using large population-scale modelling that produces insights that can drive individual-level attitudinal and behavioural change.”
*Photo by Katie Rodriguez on Unsplash