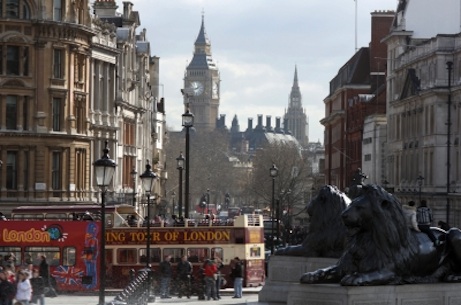
Amy Zhang leads research on an online tool which uses data compiled from social media to create real-time neighbourhood profiles.
Researchers have developed an online tool for using data compiled from social media to create real-time neighbourhood profiles which can be used to recommend specific areas to tourists based on their interests.
The research is published in a paper in the Academy of Science and Engineering HUMAN Journal. Gates Cambridge Alumna Amy Zhang is first author and presented the findings at the ASE/Institute of Electrical and Electronics Engineers Social Computing Conference in Washington DC this week.
The study, Hoodsquare: Modelling and Recommending Neighbourhoods in Location-based Social Networks, looks at how to define, classify and describe neighbourhoods using data collected from social media websites that incorporate location, such as Foursquare. The researchers built an algorithm to define the boundaries of neighbourhoods in urban spaces using the characteristics they collected data for. They also built an online visualisation at hoodsquare.org and applied their results by building a neighbourhood recommendation system.
Amy [2011], who did an MPhil in Advanced Computer Science, says: “There are many benefits to using this data in addition to traditional means such as collecting census data to define neighborhoods – namely that it is much cheaper and less time-consuming. People and places are not stationary, and characteristics of areas can change within the span of a few years or less. In contrast, the US census is only done once every ten years and involves many part-time workers and often going door-to-door. By using online social network data, we can access data about geographic areas that is both granular, in real-time and enriched with comments, profiles and other semantic information. We collect our data from Foursquare check-ins pushed to public Twitter accounts, so everything we use is publicly available on the internet.
“In our paper, we define several characteristics that we can gather from our data that we believe are defining features of different neighbourhoods, mainly the tourist level of an area, the time of day an area is busiest, and the types of venues that are within an area. From there, we find for each of these features, the areas within a city that have a highly concentration of that feature, which we call ‘hotspots’. We then combine these hotspots together to find areas with many overlapping hotspots or in other words, areas with high relative homogeneity with respect to their features, which we then classify as neighbourhoods. All the information is available at hoodsquare.org. We also devise a potential application for Twitter users given a user’s ‘About me’ profile text to recommend neighbourhoods to them. Our goal here is to provide users with geographic areas constrained in size that they then can explore and connect with on their own.”
Picture credit: www.freedigitalphotos.net and Simon Howden.