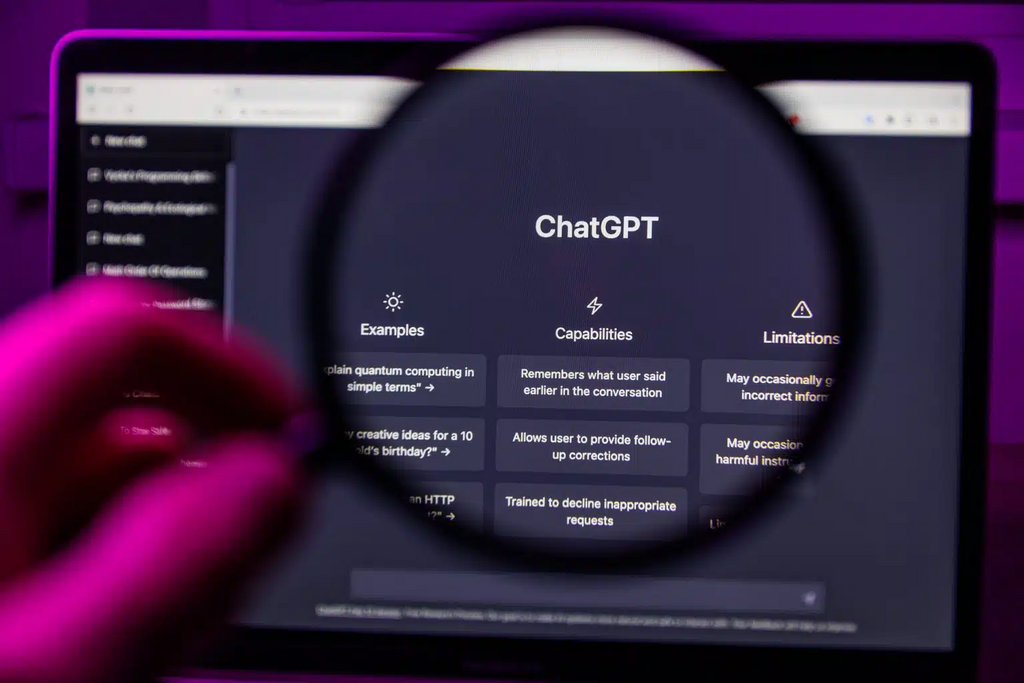
Tiancheng Hu and Yara Kyrychenko write about their new research findings on AI models and bias
As we continue developing these powerful tools, we have an opportunity - and responsibility - to ensure they don't amplify the tribal divisions that already challenge our society.
Tiancheng Hu and Yara Kyrychenko
Elections often reveal how deeply divided humanity can be. This year, as increasing polarisation continued to shape our world, we asked: Does this division transfer to our AI?
Our journey to answer this question began in 2022, when we started our PhDs as Gates Cambridge Scholars. Two concurrent events captured this moment in history: the invasion of Ukraine and the launch of ChatGPT. The political events around us highlighted a fundamental aspect of human nature – our tendency to split the world into “us” and “them” – while ChatGPT’s emergence raised new questions about whether these same tribal instincts might be embedded in our artificial systems.
Social psychologists have long studied this phenomenon through the lens of Social Identity Theory, which explains how even trivial group memberships can trigger powerful biases. When people identify with a group – be it a nation, political party, or even fans of different sport clubs – they tend to favour their own group (in-group solidarity) while viewing others more negatively (out-group hostility). This psychological mechanism underlies much of today’s polarisation.
But what about the AI systems we’re creating? Do they inherit these deeply human tendencies? “Large language models are becoming remarkably human-like in their capabilities,” explains Tiancheng. “Understanding whether they exhibit similar social biases – and potentially amplify them – is crucial as more and more people interact with them.”
In our paper, just published in Nature Computational Science, titled “Generative language models exhibit social identity biases”, we developed a method to measure these biases in large language models and tested over 70 different models.
Here’s what we found:
- Most base models – those trained on internet data and not fine-tuned to interact with everyday users- exhibit social identity biases comparable to humans – they’re about 70% more likely to speak negatively about “them” and more positively about “we”.
- Instruction-tuned models, designed to interact with humans (think chatbots like ChatGPT) and with safety guardrails, show much lower levels of bias.
- When we analysed real conversations between humans and AI, we found something surprising: these chatbots do exhibit substantial bias but human users themselves displayed more bias than the models they interacted with.
To understand where these biases originate, we trained language models on polarised data – tweets from US Democrats and Republicans. The results were striking – these models became 3-5 times more biased. But here’s the hopeful part: when we carefully filtered out extremely negative or overly tribal language from the training data, we could significantly reduce these biases.
“This shows that while AI can inherit our social biases, it doesn’t have to,” notes Yara. “We have the power to shape these systems to be more unifying than divisive.”
As AI becomes increasingly woven into the fabric of our daily lives – helping us write, make decisions and even form opinions – its biases could either amplify or help heal our social divisions. As we continue developing these powerful tools, we have an opportunity – and responsibility – to ensure they don’t amplify the tribal divisions that already challenge our society.
*Tiancheng Hu [2022] is doing his PhD in Computation, Cognition and Language. Yara Kyrychenko [2022] is doing her PhD in Psychology.
**Picture credit: Marketcomlabo and Wikimedia commons.